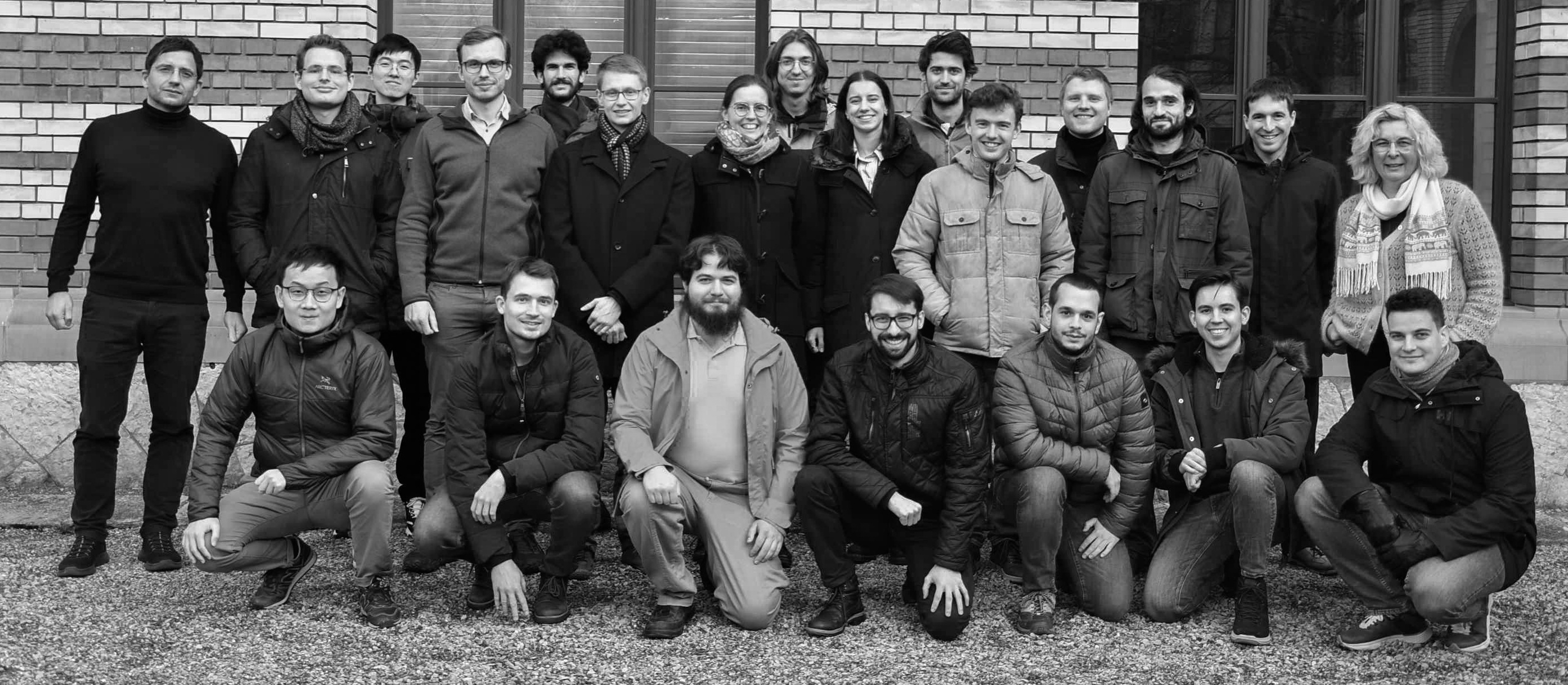
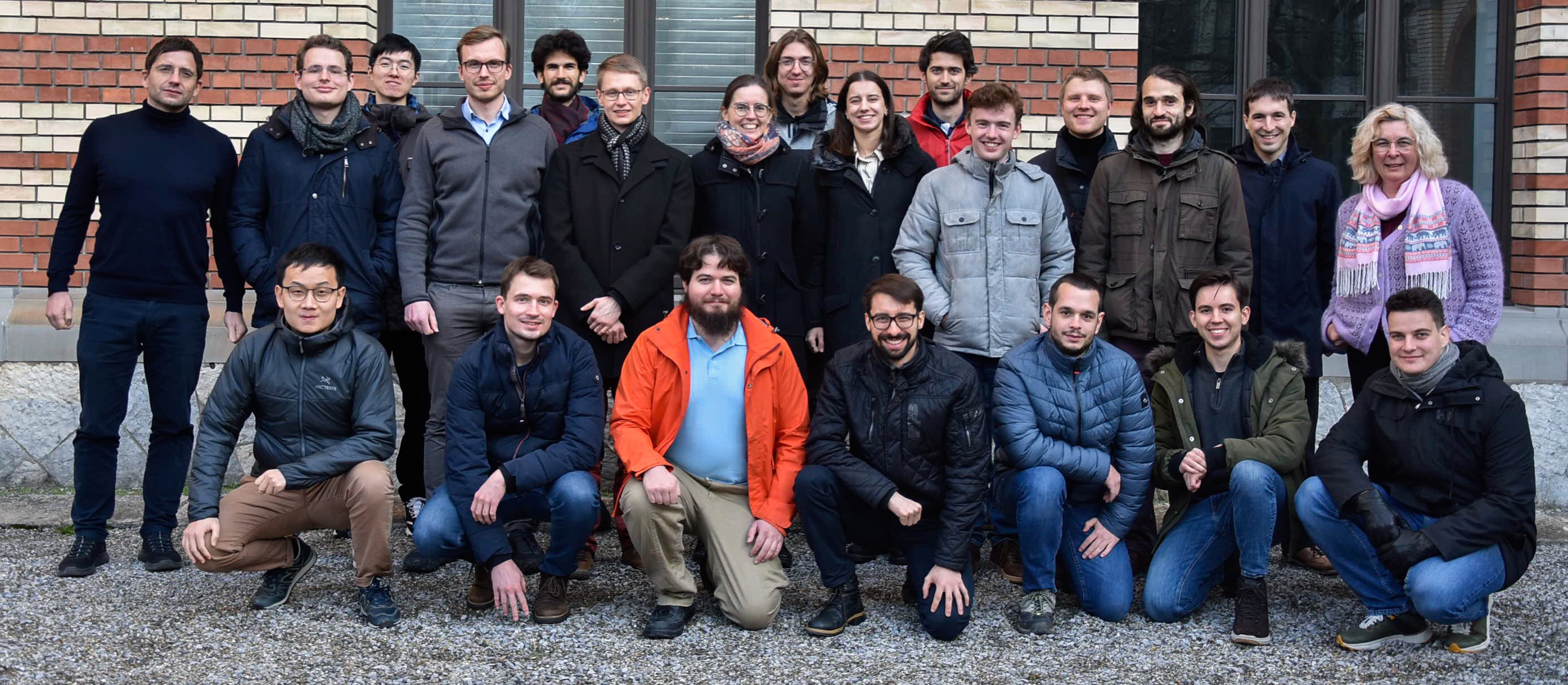
The Secure, Reliable, and Intelligent Systems (SRI) Lab is a research
group in the Department of Computer Science at ETH Zurich.
Our current research focus is on the areas of reliable, secure,
robust and fair machine learning, probabilistic and quantum
programming, and machine learning for code.
Our work led to three successful ETH spin-offs: DeepCode.ai (AI for code), ChainSecurity (security verification),
and LatticeFlow (robust machine learning).
See our Publications to learn
more about our work.
Latest Blog Posts
Latest News
Latest News & Blog Posts
The Role of Red Teaming in PETs: In February, our team won the Red Teaming category of the U.S. PETs Prize Challenge, securing a prize of 60,000 USD. In this blog post, we will provide a brief overview of the significance of Red Teaming in the field of Privacy Enhancing Technologies (PETs) research in the context of the competition.
LAMP: Extracting text from gradients with language model priors: In this work we present an attack on federated learning's privacy specific to the text domain. We show that federated learning in the text domain can expose a lot of user data.
Reliability guarantees on private data: We present Phoenix (CCS '22), the first system for privacy-preserving neural network inference with robustness and fairness guarantees.
Why tighter convex relaxations harm certified training: We investigate a long-standing paradox in the field of certified training, identifying previously overlooked properties of convex relaxations which affect training success.